Youtube offers the opportunity to access to very interesting videos, some of them may have a key role in the individual development. The enormous available choice allows to find the best match between individual necessity and individual preferences. A user can find answers presented in a form that makes the user comfortable. This match results in steepening the learning curve, a quicker learn because is also fun. From this point of view, Youtube is potentially an expert creating fabric. A full-time school for everybody, everywhere, any time.
There is also another aspect of Youtube videos to take in serious consideration, users’ interaction, this aspect adds enormous value to the main product, the video.
An individual may share opinions, critics and even feelings with other individuals by means of comments and replies. When a video is able to generate users interaction then it becomes a true lecture, an intellectual product that enriches the life of people. Moreover it could result in forming new ties among people.
Sometime in a cinema theatre the experience of looking at the people rather than the movie itself could be particularly pregnant. The observer has the possibility to rate the movie through the people’s posture and expressions.
Users’ comments and users’ likes have the same effect. An individual has the possibility to decide whether to watch the Youtube video or not by reading the users’ reactions first.
The real difference is in timing. Nobody can be blamed for being late. Youtube video may be watched any time, with or without physical company. It normally happens that individuals watch the video physically alone, but there is no loneliness in doing so. Individual watching a video as soon as decides to comment it, opens a door to users interaction, at that precise time individual becomes a user.
By commenting it starts the first interaction with the producer of the video, or the person behind the organisation that produced the video. Other users may reply to the comment. It could happen that even the producer replies. Comments, replies, replies, comments, a network arises, then suddenly a lot of facts happen. Those facts can be seen any time after they happened, everything is recorded, all the remains are still there, visiting gives a sense of archaeologic discovery, with the difference that all the actors are still alive and active.
Analyse the network that grew it is not just fascinating but also useful and functional to critical analysis of the video, to prediction for future videos, evaluation of the impact, and a lot of more.
The typical shape of the network just born, few moments after the publication of the video, is called “star”, star shaped networks indicate a one way relation, from the user to the producer.
See an example in the next figure. The circle indicates a user, connecting lines generally known as “edges” indicate a comment or a reply, in this case there are just comments.
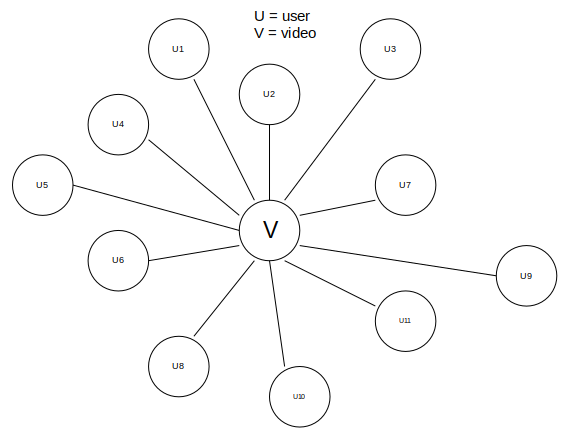
This shapes informs about a poor interaction among users and a considerable users’ interest for the video.
Star shape provides direct information about users’ opinion therefore the critical engagement.
In any case, good or bad reaction, the video has been able to start a discussion, it is the proof of an intrinsic value of the video.
Depending on the content of the comments, the value is emotional, intellectual or both.
This diagram has no direction for simplicity, but evidently the directions are from users to producer.
Let see real examples in order to understand how far can go a network analysis. Titles, urls, names or any other information that can disclose the producer and users are obfuscated for complying with legislations that protect the privacy.
The next figure shows the network created by a Youtube video with 80 users and 161 edges.
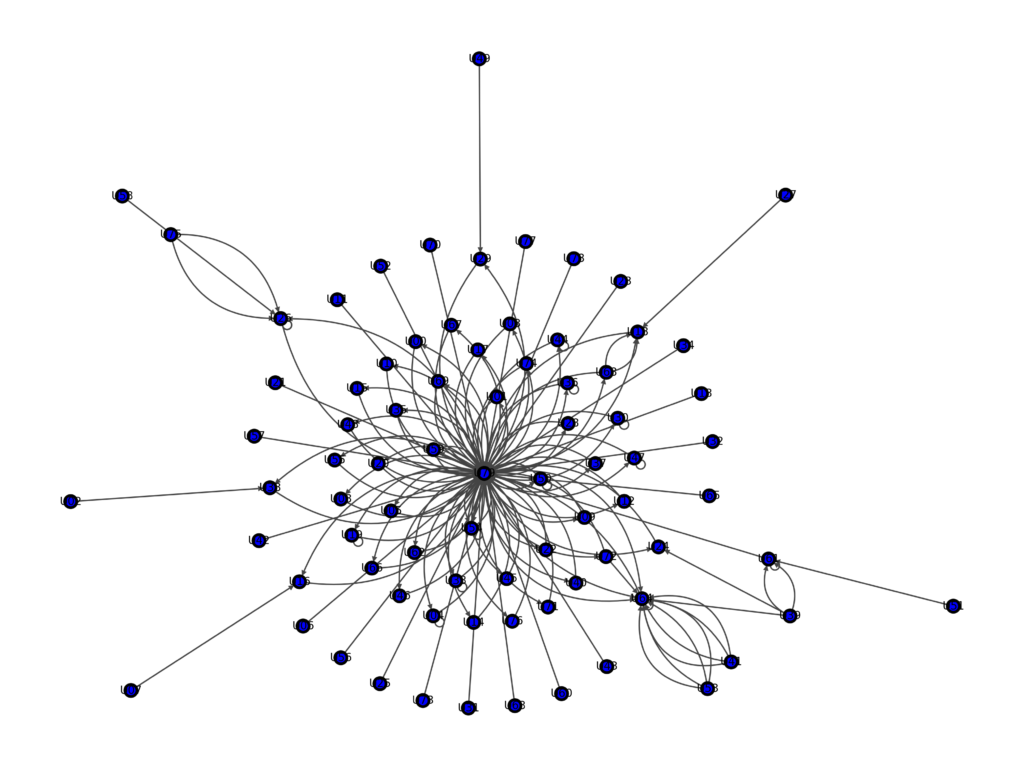
Users’ replies often create subgroups. These are a sort of subset of users discussing specific details on aspects of the content of the video.
The star shape is still visible with added subsets.
This graph informs about the level of engagement. Some users are clearly more involved than others.
It could be useful to highlight those users with a bigger level of involvement for further involvement in future videos. See the same graph with involved users highlighted in the next figure.
Users with a higher level of involvement are highlighted in red circles.
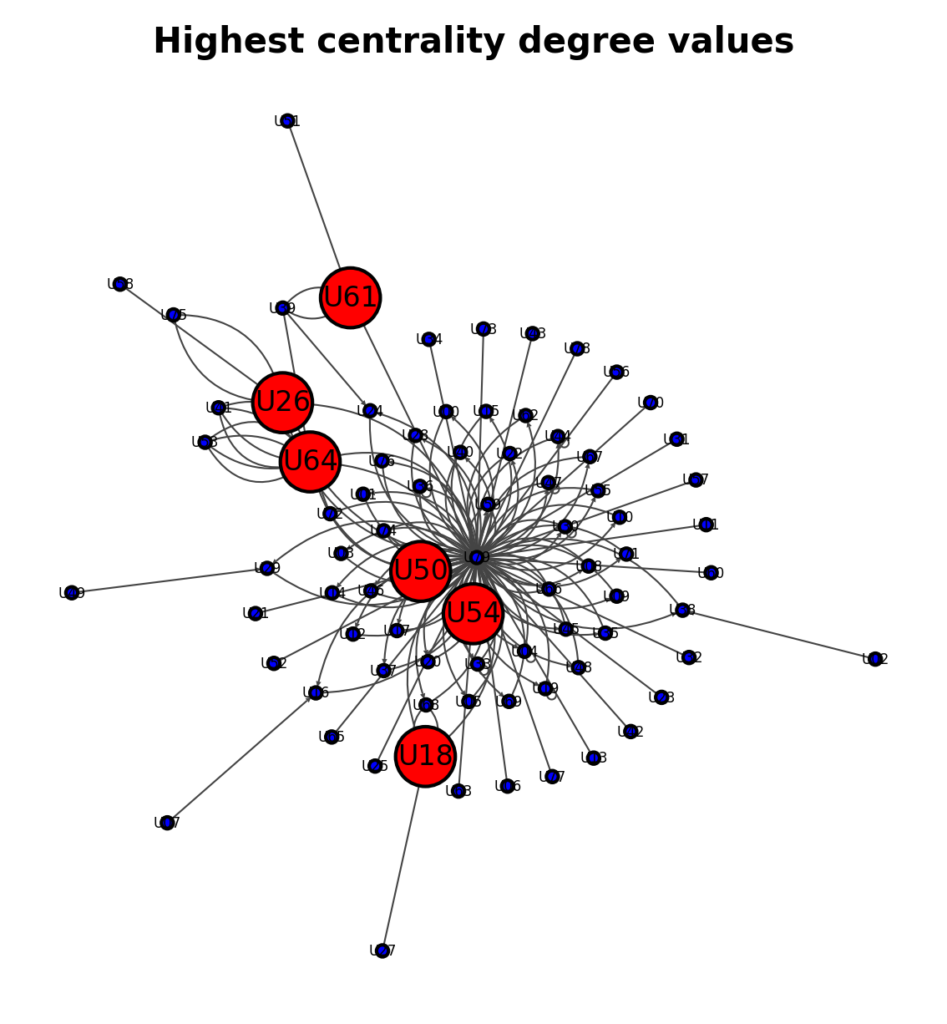
In network theory, network centrality degree is a measure of the importance or centrality of a node (or vertex) within a network. The degree of a node is simply the number of connections or edges it has with other nodes in the network. In other words, it measures how many direct links a node has to other nodes in the network.
According to the above definition, figure 3 displays those users that dispose of the higher number of replies in relation to the other users. In the graph users are nodes or vertex, comments or replies are edges.
Nodes with a high degree centrality are often considered to be more important or influential within the network, as they have more connections and are thus more likely to be able to access information and resources in the network.
Degree centrality is a simple and commonly used measure of network centrality, but it doesn’t take into account the quality or strength of the connections between nodes, and may not always be the most appropriate measure of centrality for every type of network. Because of this reason it is useful to proceed with another important network measure, hub and authorities. In order to introduce these measures is necessary to explain the role of weights in a network. In the specific case of comments and replays to a Youtube video, weights are represented by the value of “likes”.
The network weighted centrality degree is a measure of the importance or centrality of a node (or vertex) within a network that takes into account the strength or weight of the connections between nodes. Unlike the degree centrality, which only considers the number of direct connections a node has, weighted centrality takes into account the strength of those connections. Weighted centrality measures can provide a more accurate representation of the importance of a node within a network by giving more weight to nodes that have stronger or more influential connections.
The next figure shows all hubs in the network created by comments and replies to the same Youtube video referred by the previous graphs.
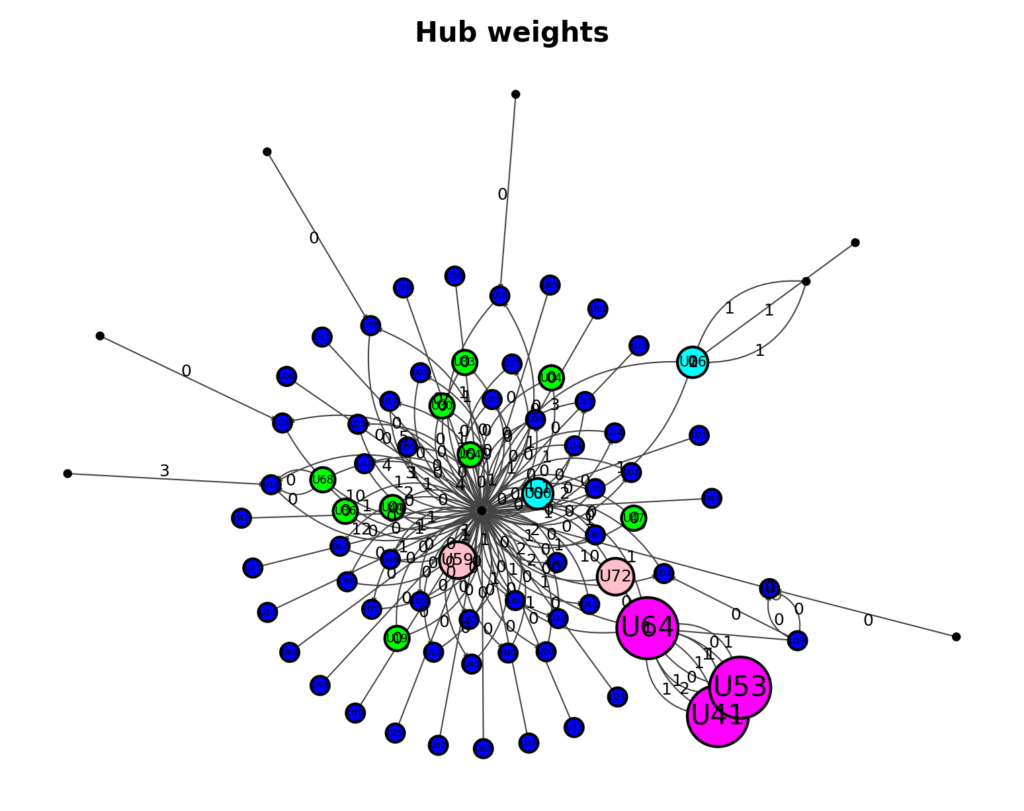
Figure 4 shows how new users emerge from a network where the number of likes are taken into account for centrality calculations. The specific calculated values are known with the name of “hubs”.
The importance of hubs in a network can be seen in their ability to facilitate communication, increase connectivity, and control the flow of resources and information within the network. Understanding the role of hubs within a network can be useful for identifying key players in a social network.
In order to understand the next centrality measure, named “authority”, the next figure visually explains the difference between a hub and an authority.
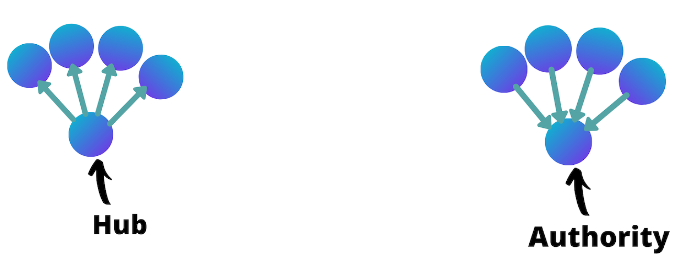
According to figure five a hub is a node that has edges pointing out of it. You can also think of a hub as a node that’s the starting node of edges. An authority, on the other hand, is a node that has edges pointing to it. You can also think of authority as a node that is the ending node of edges.
Now that the difference between hubs and authorities is clear, is possible to display the graph of authorities for the same video that previous graphs refer.
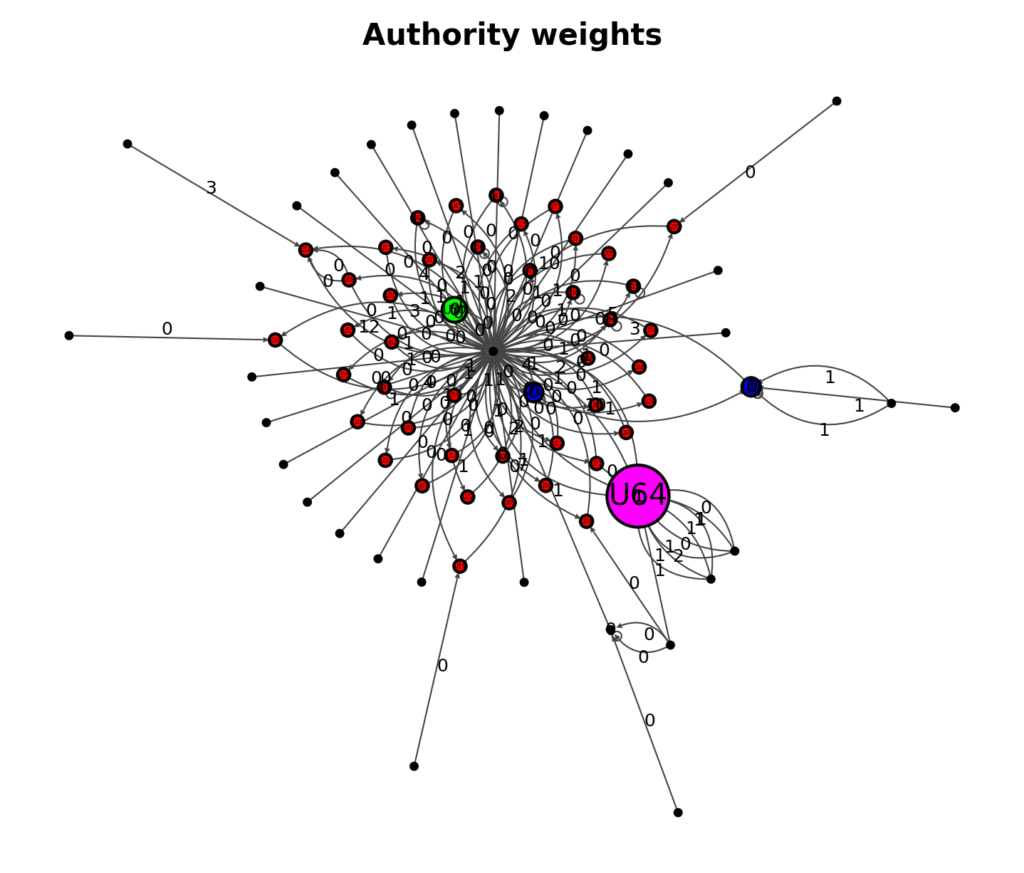
Note how in the figure six fewer users are highlighted, in particular one user.
There are plenty of different measures that can be calculated in a network, the few of them explained in this article allow to understand how some users may be recognised as having special importance in the development of the network shape and dynamics.
The video referenced up to here created a simple network because of relatively few users participating in comments and replies. With the aim of explaining the next important concept in network analysis (communities) is better to refer to a different video that had a higher number of users participating, creating, as results, a more complex graph.
The next figure represents the network graph of the mentioned video.
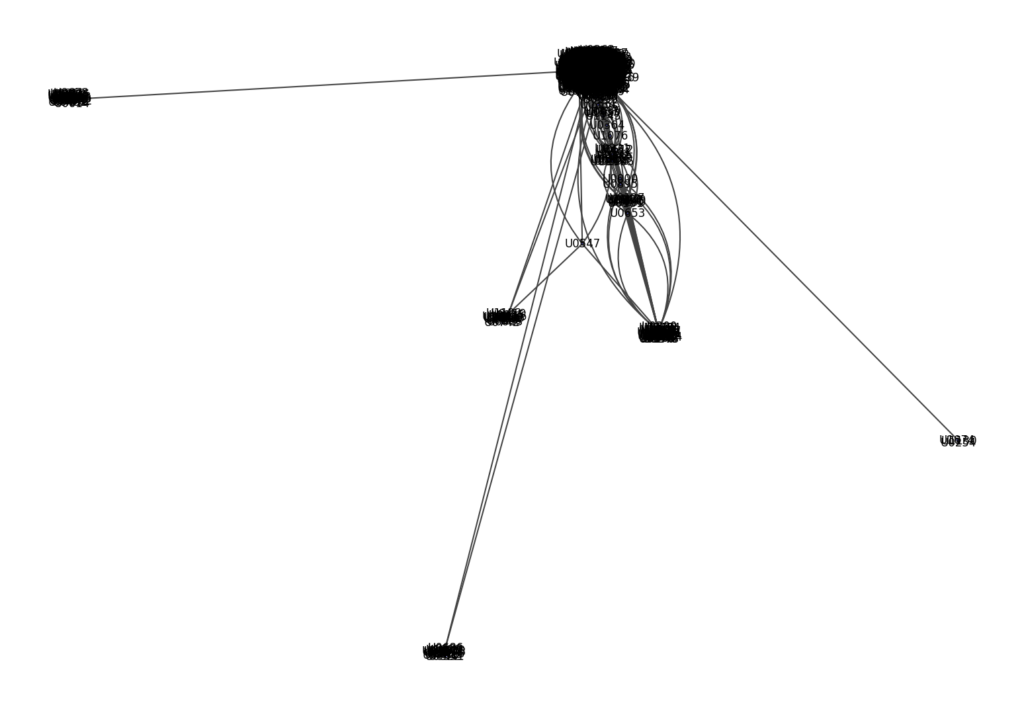
Figure seven shows a more complex network for two main reasons: a higher number of users; the video’s topic generated more discussions.
From a larger number of users and a bigger dynamic all centrality measure explained in this article may be calculated, moreover, the calculation of communities, also none as clusters, is more significant.
This video counted with the participation of 1,174 users generating 2,070 edges.
More than 20 communities where also generated. The next figure shows the larger community with 140 members.
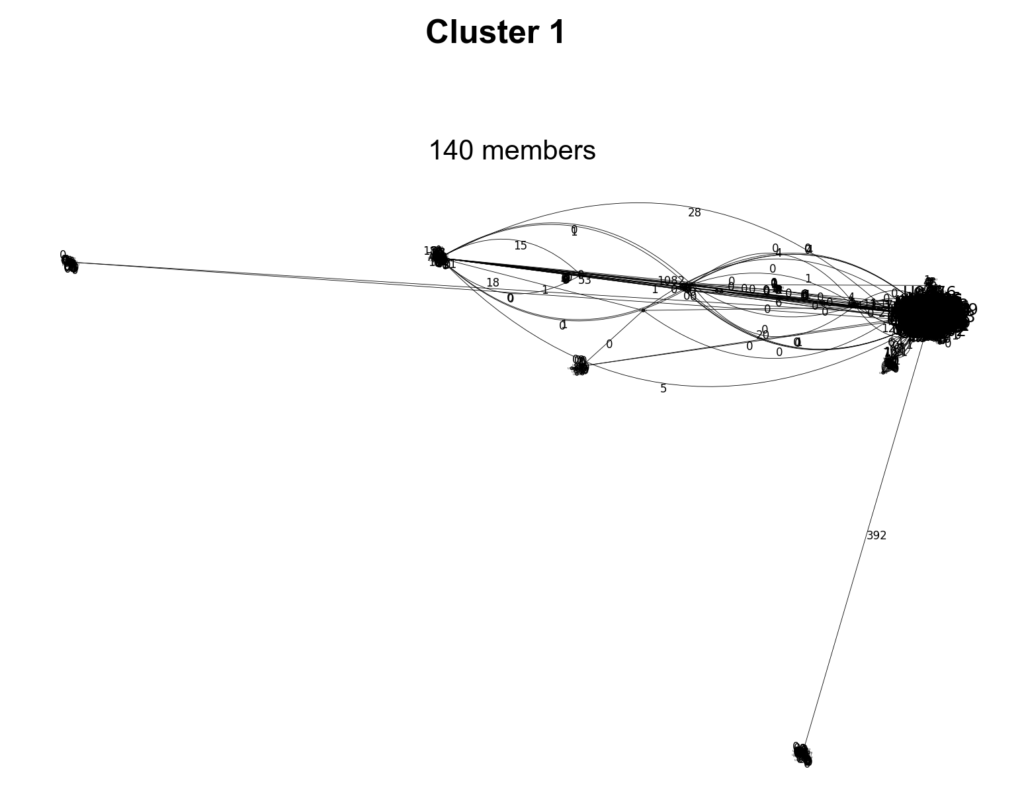
In social network analysis, community refer to groups of nodes within a network that are densely connected to one another but have relatively few connections to nodes outside the group. Communities are important in social network analysis for several reasons:
1) Community can provide insight into the structure of a network and help identify subgroups or communities within the network. By identifying communities, the analysis can better understand how different nodes are connected and the degree to which they interact with one another. This can be useful for understanding social dynamics, identifying potential sources of conflict, and identifying opportunities for collaboration or cooperation.
2) Community can provide a sense of social cohesion and social support within a network. Members of a community are often more likely to share common interests, values, and experiences, and are more likely to provide emotional support or practical assistance to one another. By identifying communities, the analysis can gain insight into the nature and strength of these social ties and can use this information to design interventions or programs that strengthen social connections and support networks.
Communities can be used to identify potential opinion leaders or change agents within a network. Members of a cluster who are well-connected within their group but also have connections to other community or nodes outside the group may be particularly influential within the network, as they are able to bridge different social groups and facilitate the spread of ideas or information.
Overall, the identification and analysis of communities in social network analysis is an important tool for understanding the structure, dynamics, and functioning of social networks. By understanding the role of communities within a network, the analysis can gain valuable insights into social cohesion, social support, and the flow of information and resources within the network.